Manually designing a cooling system will require a lot of iterations before reaching the absolute best one. But...
- What is the best design?
- How do we know it is the best?
In this sense, a mathematical formula must translate the end goal to objectively quantify all iterations concerning one another.
This blog post tackles some of the most important targets to include while designing the cooling system for a battery pack:
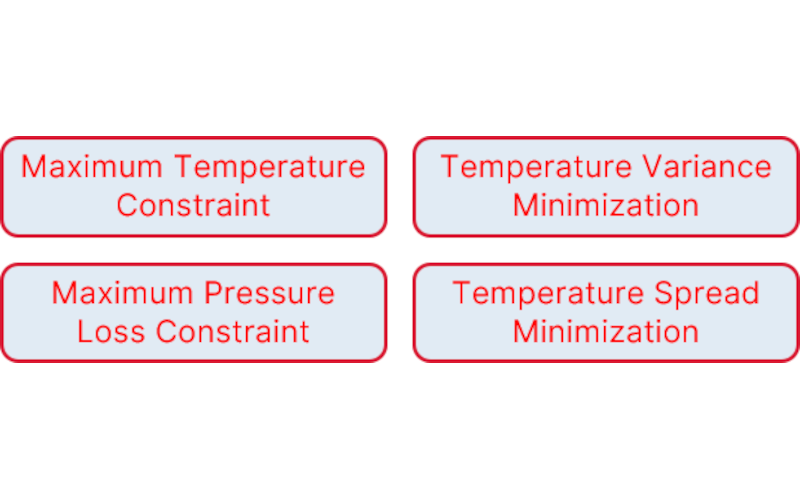
1. Maximum Temperature Constraint
The fear of any battery engineer is a thermal runaway. This phenomenon became well-known to the public when the Samsung Note 7 was released in 2016. The recall occurred after identifying a battery manufacturing defect, causing excessive heat and fires. The automotive industry also has some examples of thermal runaways, for example, GM's recall of Chevrolet Bolts.
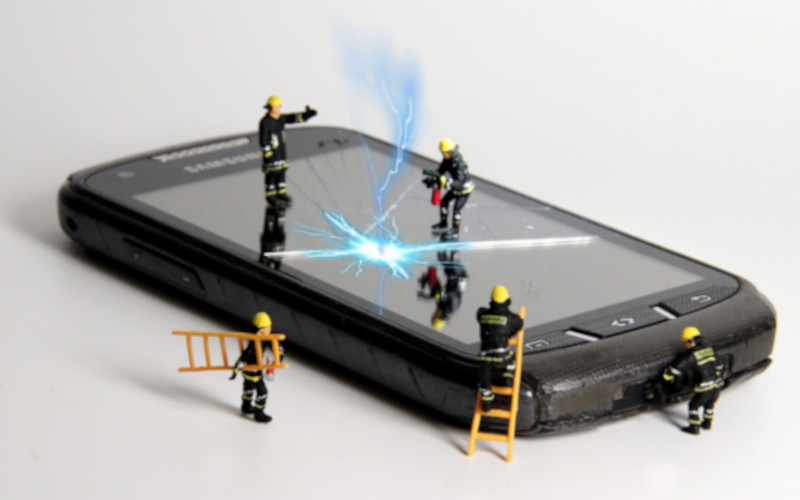
Due to the incidents mentioned above, it has become well-known that all battery packs must operate below a certain temperature limit. Going beyond this threshold value will risk thermal runaway. In the best-case scenario, thermal runaway only damages several battery cells beyond repair. In the worse case, the cells explode and start a big fire.
This threshold temperature not only makes it easy to compare the different iterations with one another but also puts a clear limit on whether or not a design is acceptable. If the maximum cell temperature exceeds the threshold, the resulting design is unsuitable for the product. Expressing this into a mathematical formula makes the idea clear:
For safety reasons, the threshold temperature will not be the temperature at which thermal runaway will commence. A safety factor (SF) will be taken into account:
2. Temperature Variance Minimization
The battery pack in EVs consists of multiple modules. Each module is subsequently done out of individual battery cells. The lifetime of the complete battery pack is thus dependent on the lifetime of each of the cells. High temperatures will consume the lifetime of the cells. Therefore, the cooling system for a battery pack is well-designed when the temperature gradient over the battery pack is limited. This fact will ensure the cells within all the modules will thermally degrade at the same pace. Thus, the goal should be to prevent the cells in one of the modules (far from the inlet) surpass their lifespan while the cells in another module (right next to the inlet) are still only halfway through their lifetime.
The variance is a statistical property with the following mathematical equation:
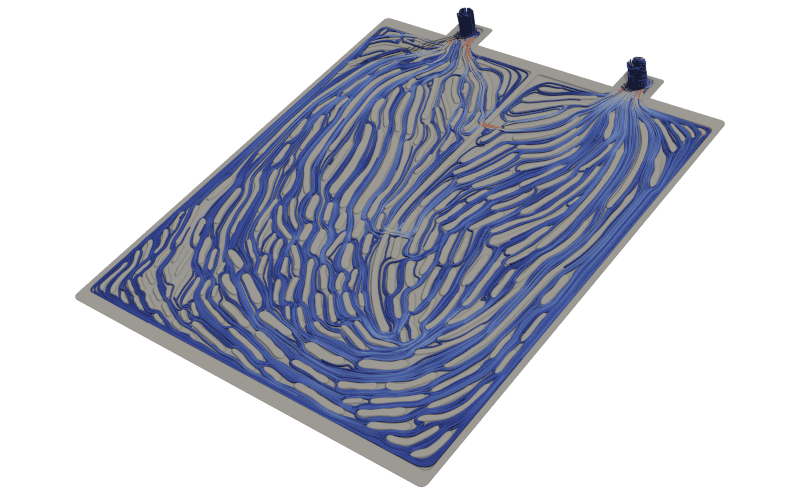
3. Temperature Spread Minimization
These requirements translate into the following penalization scheme: the further away the temperatures locally are from the optimal temperature, the more heavily these places need to get penalized during the optimization run. This penalization scheme is almost identical to the one in the previous section; only one modification needs to happen:
4. Maximum Pressure Loss Constraint
The maximum temperature constraint is not the only important constraint to consider during the design phase. The design can have an incredibly good thermal performance, but it needs to be practical. For instance, if no pump is available to force the fluid flow rate through the channels, this usually wastes engineering time, and no value is added to the product. The same holds if the pumping power required is impractically high. The energy stored in the batteries should go as much as possible to extend the EV range, not circulate the pump's required fluid.
Conclusion
Designing the cooling system for a battery pack is a complex process in which many parameters play an important role. Many iterations are needed to come up with the best possible cooling design.
The maximum temperature and pressure constraints clearly limit whether a design is acceptable or not. If a critical limit exceeds, the resulting design is useless (no matter how good the other parameters are). The temperature variance or temperature spread objectives allow for easy comparison of the different designs when both mentioned constraints are met. The design with the lowest value is the best-performing one.
The design process is complex and requires a lot of expertise to critically analyze each iteration's performance. Luckily, the generative design tool, Diabatix's ColdStream, fully automates this process in an approachable way. If you want to know more about this tool, don't hesitate to contact us. And if you would like to know more about other targets, feel free to consult our documentation.